door Eelco van Tilborg | apr 15, 2025 | Software development
Be Taught the way to efficiently practice your Natural Language Understanding (NLU) mannequin with these 10 easy steps. The article emphasises the importance of training your chatbot for its success and explores the distinction between NLU and Pure Language Processing (NLP). It covers crucial NLU components such as intents, phrases, entities, and variables, outlining their roles in language comprehension. The coaching course of involves compiling a dataset of language examples, fine-tuning, and expanding the dataset over time to improve the model’s efficiency. Finest practices include starting with a preliminary analysis, making certain intents and entities are distinct, using predefined entities, and avoiding overcomplicated phrases.
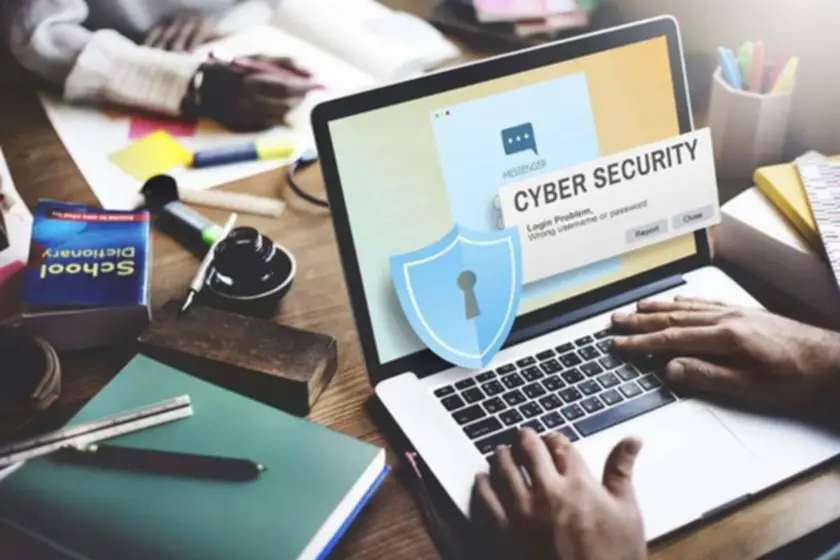
A frequent false impression is that synonyms are a method https://www.globalcloudteam.com/ of bettering entity extraction. In fact, synonyms are more closely related to data normalization, or entity mapping. Synonyms convert the entity value provided by the person to another value-usually a format wanted by backend code. If you may have added new customized knowledge to a model that has already been skilled, additional training is required.
What’s Nlu And Why Is It Essential For Beginners In Ai?
This allows engineers to establish which parameters and KPIs to retailer within the vehicle datasets that shall be used for AI coaching. Whereas NLU selection is necessary, the data is being fed in will make or break your model. This appears cleaner now, however we have modified how are conversational assistant behaves! Generally once we notice that our NLU mannequin is damaged we’ve to vary each the NLU mannequin and the conversational design. It’s important to keep in mind that models usually are not static and require continuous updates with new information to enhance their accuracy and enable them to deal with new situations. If you have a messy data set, it could be higher to begin from scratch, and assess your knowledge primarily based on the best practices listed above.
You see, before including any intents, entities, or variables to your bot-building platform, it’s usually wise to record the actions your customers may want the bot to perform for them. Brainstorming like this allows you to cover all necessary bases, while additionally laying the foundation for later optimisation. Simply don’t slender the scope of these actions an excessive quantity of, in any other case you danger overfitting (more on that later). From the list of phrases, you additionally define entities, corresponding to a “pizza_type” entity that captures the several sorts of pizza clients can order. Instead of listing all possible pizza varieties, merely outline the entity and supply sample values. This method permits the NLU mannequin to know and process user inputs precisely with out you having to manually listing each potential pizza type one after another.
Right Here, inputs represent the enter options, and targets are the corresponding output values we wish the network to learn to predict. A higher confidence interval will allow you to be more certain that a consumer says is what they imply. The draw back is that the consumer may need to repeat themselves which ends up in a irritating expertise. The different is to set a decrease value and doubtlessly direct the person down an unintended path. Our different two choices, deleting and creating a brand new intent, give us more flexibility to re-arrange our data primarily based on consumer wants. We can see an issue off the bat, each the verify balance and manage credit card intent have a steadiness checker for the credit score card!
- Real-world NLU purposes corresponding to chatbots, customer help automation, sentiment analysis, and social media monitoring have been additionally explored.
- If that is your goal, the most fitted choice is to provide coaching examples that embody commonly used word variations.
- This information provided an outline of well-liked NLU frameworks and instruments like Google Cloud NLU, Microsoft LUIS, and Rasa NLU to assist get started with growth.
- By passing nlu.md file to the above perform, the training_data gets extracted.
- So when someone says “hospital” or “hospitals” we use a synonym to transform that entity to rbry-mqwu before we move it to the customized motion that makes the API call.
To prepare an efficient NLU model, start by amassing a wide selection of knowledge that reflects different regions, languages, and person demographics. If you’re focusing on lead era, look for information sources that provide insights into consumer intent and conduct. Your intents should operate as a collection of funnels, one for every motion, however the entities downstream should be like nice mesh sieves, specializing in specific pieces of data.
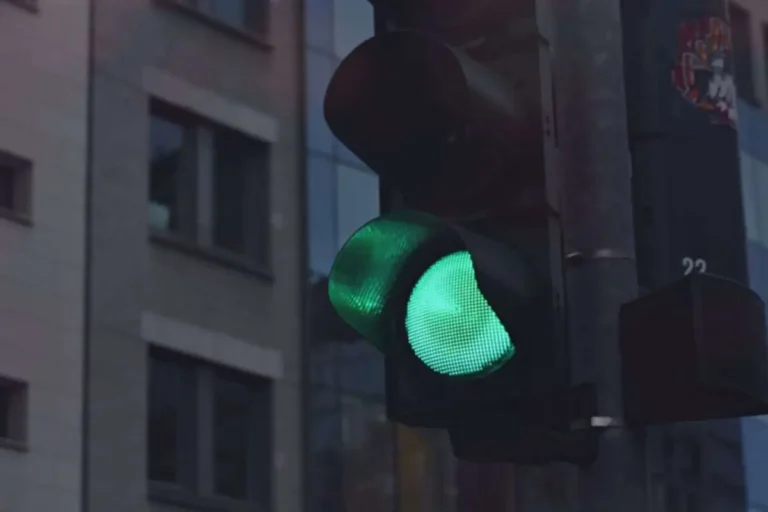
With higher information balance, your NLU ought to have the ability to be taught higher patterns to acknowledge the variations between utterances. In the past section we lined one instance of bad NLU design of utterance overlap, and in this part we’ll discuss good NLU practices. Likewise in conversational design, activating a sure intent leads a user down a path, and if it’s the “wrong” path, it’s usually extra cumbersome to navigate the a UI. We must be cautious in our NLU designs, and while this spills into the the conversational design house, excited about consumer behaviour is still elementary to good NLU design. These are the anticipated user commands and also what the mannequin will study through the training course of.
Logistic Regression – A Complete Tutorial With Examples In R
That is why information scientists often spend more than 70% of their time on data processing. It’s a on condition that the messages users send to your assistant will comprise spelling errors-that’s simply life. Many builders try to tackle this drawback utilizing a customized spellchecker component in their NLU pipeline. But we’d argue that your first line of defense in opposition to spelling errors ought to be your training information. If you have inherited a very messy data set, it might be better to begin out from scratch. But if things aren’t quite so dire, you can start by removing training examples that do not make sense and then build up new examples based on what you see in real life.
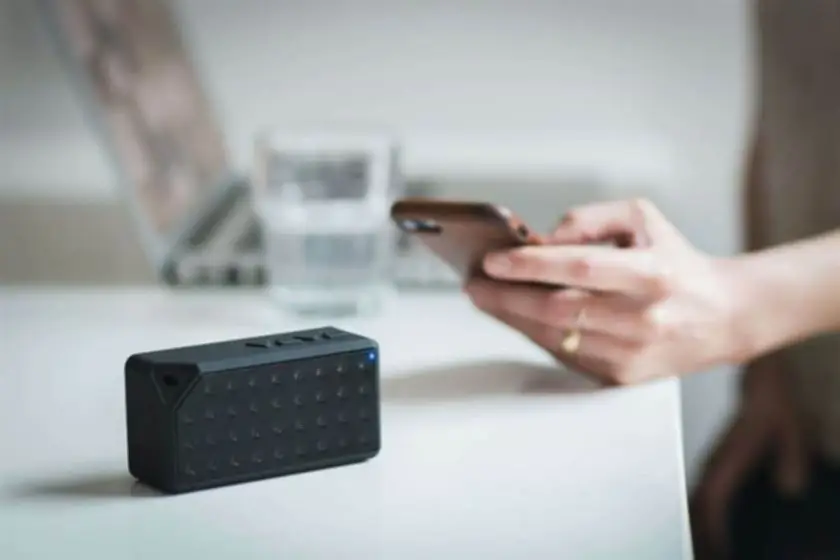
These are the actions that the user needs to perform with the system. If you keep these two, avoid defining begin, activate, or comparable intents as properly as, as a outcome of not only your model but in addition people will confuse them with start. To return a response primarily based on the detected intent you’ll have the ability to create a response dictionary or fetch responses from a DB immediately. In the subsequent article, I will train a RASA mannequin ( NLU + CORE ) which could have the aptitude to return responses itself.
It offers pre-trained fashions for many languages and a easy API to incorporate NLU into your apps. A well-liked open-source natural language processing package deal, spaCy has solid entity recognition, tokenization, and part-of-speech tagging capabilities. Pre-trained NLU fashions are fashions already educated on huge quantities of data and able to general best nlu software language understanding.
How Do You Evaluate The Efficiency Of An Nlu Model?
In the next set of articles, we’ll focus on the way to optimize your NLU using a NLU supervisor. Google Cloud NLU is a powerful tool that offers a spread of NLU capabilities, including entity recognition, sentiment evaluation, and content classification. You can use strategies like Conditional Random Fields (CRF) or Hidden Markov Fashions (HMM) for entity extraction. These algorithms take into account the context and dependencies between words to identify and extract particular entities talked about within the textual content. The answer is a hybrid resolution that mixes check and simulation to make accurate predictions at the full vehicle level and enable the era of large datasets.
Datasets grow over time so to take care of their worth they should be successfully managed. Simcenter Testlab Workflow Automation (TWA) accounts for this dynamic character of the dataset by automating the retrieval, processing, and publishing of data as soon as it’s gathered. This ensures that when new knowledge is added to the dataset, AI tools are retrained with it and frequently enhance their accuracy. In the case the place the skilled dataset indicates a low degree of prediction accuracy, the AI software offers suggestions on what knowledge it needs to improve.
Often it is mixed with ASR in a model that receives audio as enter and outputs structured textual content or, in some instances, utility code like an SQL query or API name. This combined task is often known as AI Robotics spoken language understanding, or SLU. When a person sends a message, it’s handed by way of the NLU pipeline of Rasa.
door Eelco van Tilborg | apr 15, 2025 | Software development
Merchants can combine with Danger SDK to seize device knowledge like geolocation, IP address, and system fingerprinting, which are then analyzed using machine studying models to evaluate fraud threat in real time. The rise in digital transactions has inevitably heightened the risk of fraud, significantly for on-line or card-not-present (CNP) transactions. In response to this growing threat, the integration of AI and ML has emerged as a compelling answer. Artificial intelligence is becoming broadly used in cost processing to enhance efficiency, security, and buyer experience.
Schedule a demo at present to see how AI can accelerate and rework your digital payments. In this article, we’ll discover how AI impacts digital payment practices, the expertise concerned, its uses, advantages, and challenges of AI in funds. As the world strikes to additional improvements like real-time payments in 2024, AI’s at-speed capabilities will become more critical around orchestration and fraud protection.
For instance, in the banking sector, AI’s analytical prowess allows for a complete evaluation of a buyer’s cost and transaction historical past. This evaluation leads to tailor-made offerings, including personalised loans and credit card decisions, full with loyalty programmes that resonate with the individual customer’s preferences. The AI software, while extremely efficient, is not infallible, and it could often generate false positives (flagging a reliable transaction as fraudulent) or false negatives (missing precise fraudulent activities). These errors can end result in financial losses, harm a business’s backside line, erode buyer trust, and tarnish the general buyer experience. Finding the proper balance between minimising false positives and false negatives is important, and attaining this will likely involve incorporating human assets and experience.
The Transformative Power Of Cx In Banking
The introduction of credit and debit cards was a leap toward convenience and safety. Playing Cards eased the burden of carrying money and offered a traceable, straightforward method to transact. They grew to become particularly popular with the introduction of level of sale (POS) expertise, which facilitated straightforward payments in bodily retail settings. The world of commerce has undergone radical transformations over the many years, transferring from a primarily cash-based system to one that embraces digital know-how at its core. Let’s take a journey through the evolution of fee methods to know why the search for better, faster, and safer fee choices is essential.
Payments As A Service
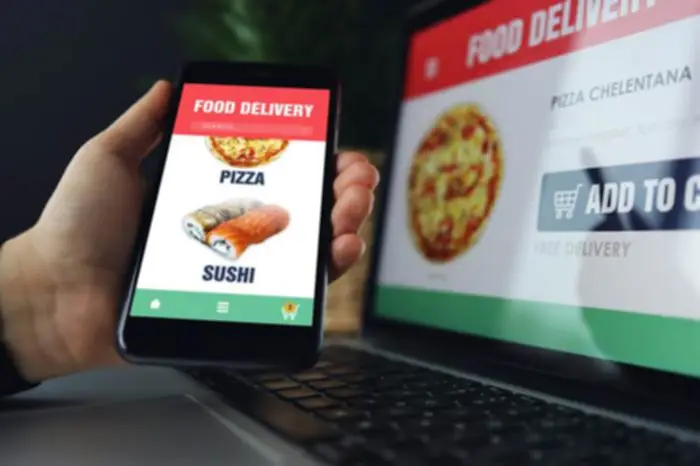
Human-AI collaboration would require abilities corresponding to critical considering, strategy and regulatory interpretation to supervise AI-driven insights. AI strengthens safety measures by figuring out vulnerabilities and potential threats. Nonetheless, implementing AI includes building the information and technical infrastructure to accommodate the expertise https://www.globalcloudteam.com/. These steps are neither simple nor cheap, and organizations must align their workforces to turn out to be proficient in offering context to AI models.
Banks like Financial Institution of America use AI-driven chatbots to help prospects with inquiries, transaction history genai in payments, and even monetary advice. These chatbots present quick, correct responses, enhancing buyer satisfaction and reducing the workload on human customer service agents. AI improves the customer expertise by tailoring suggestions and presents primarily based on individual conduct and preferences. This personalization enhances buyer satisfaction and loyalty, as users obtain related and customized interactions that meet their particular needs. Blockchain expertise offers a decentralized and safe ledger for recording transactions.
- AI algorithms analyse transaction information to establish anomalies, corresponding to unusual spending behaviour or system utilization.
- AI helps tailor recommendations and provides primarily based on buyer behavior and preferences.
- From retail giants to budding fintech startups to small to medium-sized enterprises (SMEs), the ripple impact of AI in payments is felt throughout industries.
- Past threat administration and customer service, AI will increasingly optimize back-end operations around processing, reconciliation, and settlements.
AI is right for expediting fee tasks and processes, given its capabilities in smart decision-making despite high ranges of complexity. Machine learning can serve payment processing with optimal cost routing, value optimization, and complicated fraud detection. Intelligent Acceptance implements multiple optimizations throughout the entire fee move. This increases the chance that the issuer will authorize fee requests on the primary attempt, facilitating easy cost experiences for patrons. AI can improve authorization rates through performing optimizations on fee requests and retries. Clever Acceptance is Checkout.com’s machine studying engine for fee processing; it’s informed by data from billions of fee makes an attempt, and regularly analyzes and adapts funds to improve auth charges.
This combination results in extra efficient IoT operations, better interactions between people and machines, and improved information administration and analysis. Despite this, incorporating AI in payments techniques introduces important regulatory issues. Monetary institutions must establish an AI strategy that defines acceptable practices, incorporates pre-launch evaluations, and maintains ongoing compliance checks. For instance, it might possibly deal with tasks such as invoice processing, knowledge entry, and transaction categorisation, which historically consume plenty of effort and time. AI-enabled machine learning instruments refine the accuracy of transaction approvals by detecting authentic transaction patterns. It slashes false positives and negatives to make sure legitimate transactions aren’t wrongly declined.
AI-powered invoice processing can minimize errors in knowledge enter, and expedite funds with timely reminders. This not solely accelerates funds but also improves payment data accuracy, fostering stronger business relationships. If you’re taking payments within the European Economic Space, then Strong Buyer Authentication is a must.
Leveraging data offers businesses the ability to raised understand buyer behaviors, preferences, ache points, and purchase drivers. To maximize value, companies ought to search partners who provide actionable insights that drive measurable results. Clear, structured information not only improves effectivity, but in addition serves as a springboard for delivering exceptional payment experiences. It struggled to comprehend my issue – and persistently offered the same chilly and impersonal responses. Frustrated, I in the end selected to call customer support, enduring a wait of over half an hour to speak with a consultant.
If their bill isn’t due but, they might be on the lookout for information corresponding to their payoff date or account details. AI will automate most of the handbook duties concerned in reconciliation, similar to data entry, matching, and exception dealing with. This will help reduce errors and enhance the speed and accuracy of the reconciliation process.
With their pure language interfaces, spectacular output and skill to wrangle massive, unstructured datasets, gen-AI tools have caught the imagination. They not only characterize a new, conversational method to interact with machines, however a means for machines to perform duties that were previously thought to be the protect of humans. This has spurred the digital economy to embrace gen-AI with gusto, prompting entrepreneurs to launch new startups, and tech giants to quickly introduce new software program options. Offering funding banking options, including mergers and acquisitions, capital elevating and danger administration, for a broad vary of corporations, institutions and governments.
If you’re nonetheless handling these processes manually, you’re missing out on serious efficiencies. The funds industry has seen many changes in current years, with the rise of cell funds and digital wallets. Nevertheless, one big change in the payments industry is more probably to come from synthetic intelligence (AI). With the superb capabilities of Synthetic Intelligence fashions and how top digital cost firms are making use of them, it’s solely a matter of time earlier than we see even higher use cases. By analyzing user information and identifying suspicious activities, AI can help mitigate the danger of economic crimes and ensure regulatory compliance.
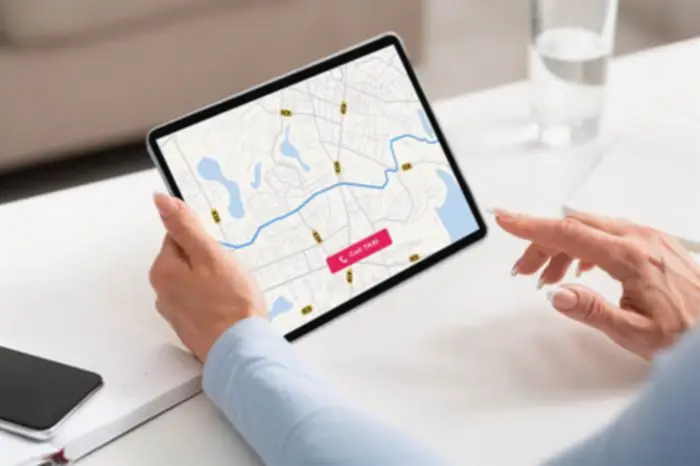
Tips On How To Construct An App Like E& Money?
In the complicated, dynamic world of payments, guaranteeing clean, secure, and quick transactions is a excessive concern for cost service providers, retailers, financial establishments, and customers. In a world that’s quickly evolving toward digitalization, the need for seamless, efficient, and safe transactions is significant. The financial ecosystem is experiencing a transformative section with the incorporation of artificial intelligence (AI) in payment methods. AI improves cost reconciliation by automating matching invoices with payments, detecting discrepancies, and rapidly flagging errors. Over time, machine studying algorithms refine accuracy by learning from information patterns. Leveraging AI, fee firms are producing real-time insights from large transaction data stores.
Conversational AI helps payment corporations tackle a extensive range of frequent buyer queries without human intervention. Clients can now get prompt assistance regarding account balances, cost due dates, transaction disputes, and other points by simply having a dialog with an AI assistant. This has substantially lowered call centre volumes whereas improving 24/7 support accessibility. According to a report by Mordor Intelligence, the AI cost Front-end web development market is predicted to develop at a CAGR of over 20% between 2022 and 2027. This progress is being pushed by the power of AI to reinforce key areas of funds like fraud detection, customer support, underwriting, and more.